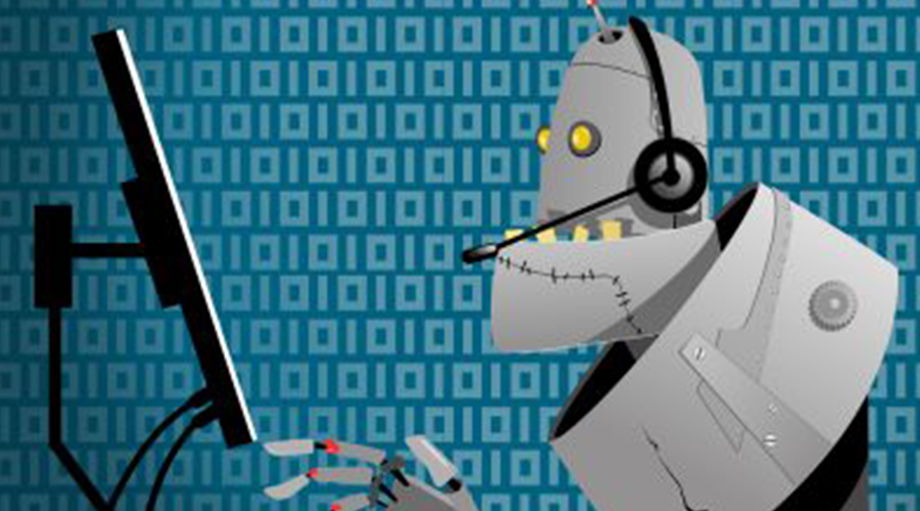
The fact that machine learning (ML) services are now available through AWS has led to a shift in the capabilities that developers can offer their clients with regards to natural language processing (NLP) and the cloud services they inform.
Machine learning is an area of artificial intelligence (AI) built on the idea that systems can learn from data, identify patterns and make decisions. NLP is a subset of auxiliary services that are defined as the automatic manipulation of natural language, like speech and text, by software.
Essentially, its machines learning to speak as we do, and it’s integrating into more processes than you thought.
Rory Preddy, part of the research and development team at software development company BBD sees these auxiliary or helper services as functionality that the everyday developer wants. “The best part is they’re accessible without having to buy-in to a much larger investment.”
How do these ML services fit into the bigger cloud picture?
“It is no secret that we’re in the middle of a cloud race, and for the exception of Google, everyone is racing to woo Africa for cloud adoption, with promised intentions to bring data centres to South Africa. As an AWS Standard CloudFront Partner, BBD’s access to these machine learning services allows us to better deliver premium ML-based cloud solutions for our clients,” explains Preddy.
So, what is NLP?
The four pertinent NLP services offered by AWS consist of Translate, Comprehend, Transcribe and Poly. Together, these services can take text to voice and vice versa, translate between languages, transcribe audio files on mass and extract insights from stacks of documents – all tasks that can now take a fraction of the time to complete.
An example of how the services work together are chatbots. Although they currently rely on text, the integrated services ensure that information could be transcribed from voice to text, allowing the bot to compute and reply. Using Poly, that response could be verbally relayed to the user interacting with the chatbot. It is in this way that NLP services are creeping into processes in unexpected ways.
What are the most common uses for NLP?
• Email filters use NLP to assess the likelihood of mail being spam
• Algorithmic trading and sport betting
• Digital conversations from chat bots
• As a compliance tool
• Pulling specific information from thousands of documents
• Possibly the number one use for NLP is for social media sentiment
Digital marketing teams make use of NLP tech to monitor online sentiment regarding their brands. This sentiment analysis is the difference between the proactive “Pull the product, they say it’s catching on fire”, and the reactive “We should have pulled the product before all the reviews did so much damage to the brand”.
But with every new use for tech comes challenges. For NLP services, the challenges revolve around conversational attributes such as ambient noise, conversational nuances and artefacts, cross-talking, code-switching (hopping between languages during a phrase), unknown words or specialised vernacular and low-resource languages and dialects. Many of these challenges are especially pertinent in the South African language environment.
Luckily, the NLP services remain true to their ML roots and while the core services continue to learn, AWS is training the software to handle exceptions and better understand the intricacies of human language. “The software will probably understand it all before I do” jokes Preddy.